ASCO 2021: Looking Toward Balancing Equity and Selectivity in Cancer Care
by Lisa Green
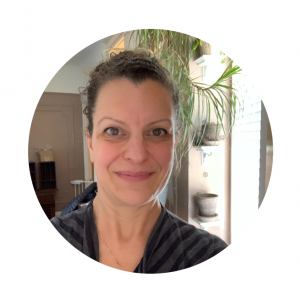
ASCO 2021 came at a very interesting time – on the cusp of “return to (new) normal” after the COVID-19 pandemic, and with an increased focus and scrutiny on diversity, equity, and inclusion. The past months have certainly required flexibility, compassion, and resilience on many levels and, even in the unprecedented environment brought on by the pandemic, commitment to evolving care for all oncology patients was evident. With a little distance since ASCO, I find myself coming back to a couple of intriguing ideas that support this essential advancement when considering patients with cancer.
The first is the idea of “right-sizing” therapy for oncology patients. Dr. Erika Hamilton from the Sarah Canon Research Institute discussed this during her presentation on new treatments for HER2+ breast cancer during the session FDA Approvals and Their Incorporation into Clinical Practice. Dr. Hamilton described “right-sizing” as “not too much, not too little treatment, tailoring for specific patient characteristics and what they need to have excellent outcomes.” Although this idea is most relevant for tumors that have a wide treatment armamentarium, it’s a potentially relevant consideration across many tumor types that aligns clinically with aspects of the movement for better cancer care for all, but not a one-size fits all approach.
Another complementary approach is the idea of using AI and advanced modeling to help best define patient selection for clinical trials based on who may receive the greatest benefit. This was highlighted by Dr. Michal Tomaszewski from Moffitt Cancer Center in his talk “Imaging-based patient inclusion model for clinical trial performance optimization” during the session Artificial Intelligence: Optimizing Cancer Care Using Imaging and Pathology. Dr. Tomaszewski asserted this approach leverages the power of machine learning patient selection algorithms to define characteristics that might help refine clinical trial patient selection through predictive models. By using standard of care information that is readily available at no cost, the resulting algorithm could be used “to assist physicians, in a cost-effective way, in making the right clinical decisions in places where clinical oncology expertise may be limited.” As Dr. Tomaszewski noted, this aspect of the approach supports the increased equity of patient selection and inclusion in trials with increased clinical selectivity based on potential patient sensitivity to a treatment.
These ideas balance the need for diversity, equity, and inclusion to make quality care available to all oncology patients with careful consideration of what is best for each patient. It will be exciting to see how the field of oncology will incorporate “right-sizing” and selection of treatments to continue the optimization of cancer care.